YOLOv5¶
Abstract¶
YOLOv5 is a family of object detection architectures and models pretrained on the COCO dataset, representing Ultralytics open-source research into future vision AI methods, incorporating lessons learned and best practices evolved over thousands of hours of research and development.
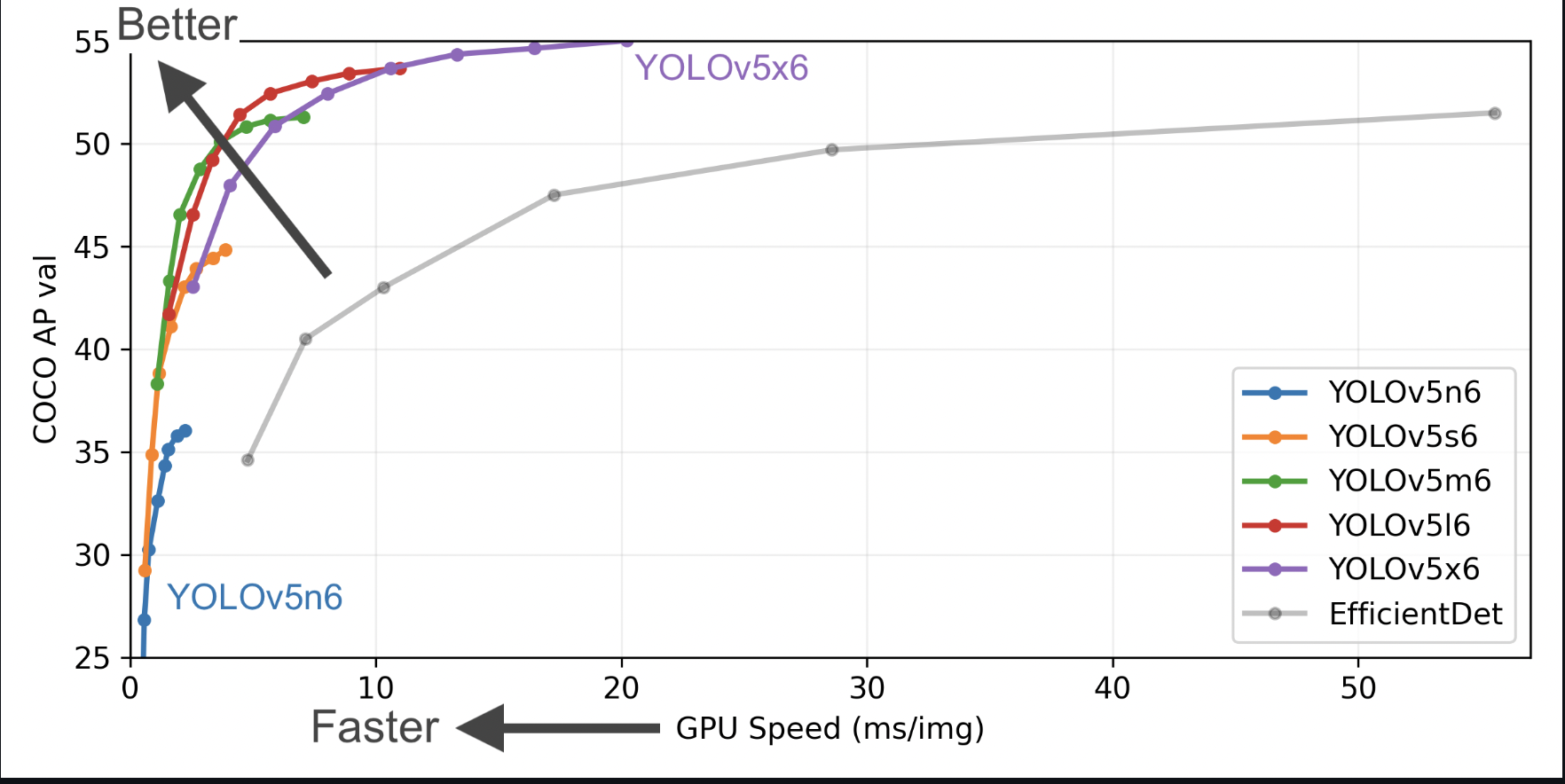
Results¶
performance tested on Ascend 910(8p) with graph mode
Name | Scale | BatchSize | ImageSize | Dataset | Box mAP (%) | Params | Recipe | Download |
---|---|---|---|---|---|---|---|---|
YOLOv5 | N | 32 * 8 | 640 | MS COCO 2017 | 27.3 | 1.9M | yaml | weights |
YOLOv5 | S | 32 * 8 | 640 | MS COCO 2017 | 37.6 | 7.2M | yaml | weights |
YOLOv5 | M | 32 * 8 | 640 | MS COCO 2017 | 44.9 | 21.2M | yaml | weights |
YOLOv5 | L | 32 * 8 | 640 | MS COCO 2017 | 48.5 | 46.5M | yaml | weights |
YOLOv5 | X | 16 * 8 | 640 | MS COCO 2017 | 50.5 | 86.7M | yaml | weights |
performance tested on Ascend 910*(8p)
Name | Scale | BatchSize | ImageSize | Dataset | Box mAP (%) | ms/step | Params | Recipe | Download |
---|---|---|---|---|---|---|---|---|---|
YOLOv5 | N | 32 * 8 | 640 | MS COCO 2017 | 27.4 | 736.08 | 1.9M | yaml | weights |
YOLOv5 | S | 32 * 8 | 640 | MS COCO 2017 | 37.6 | 787.34 | 7.2M | yaml | weights |
Notes¶
- Box mAP: Accuracy reported on the validation set.
- We refer to the official YOLOV5 to reproduce the P5 series model, and the differences are as follows: We use 8x NPU(Ascend910) for training, and the single-NPU batch size is 32. This is different from the official code.
Quick Start¶
Please refer to the QUICK START in MindYOLO for details.
Training¶
- Distributed Training¶
It is easy to reproduce the reported results with the pre-defined training recipe. For distributed training on multiple Ascend 910 devices, please run
# distributed training on multiple Ascend devices
msrun --worker_num=8 --local_worker_num=8 --bind_core=True --log_dir=./yolov5_log python train.py --config ./configs/yolov5/yolov5n.yaml --device_target Ascend --is_parallel True
Note: For more information about msrun configuration, please refer to here.
For detailed illustration of all hyper-parameters, please refer to config.py.
Note: As the global batch size (batch_size x num_devices) is an important hyper-parameter, it is recommended to keep the global batch size unchanged for reproduction or adjust the learning rate linearly to a new global batch size.
- Standalone Training¶
If you want to train or finetune the model on a smaller dataset without distributed training, please run:
# standalone training on a CPU/Ascend device
python train.py --config ./configs/yolov5/yolov5n.yaml --device_target Ascend
Validation and Test¶
To validate the accuracy of the trained model, you can use test.py
and parse the checkpoint path with --weight
.
python test.py --config ./configs/yolov5/yolov5n.yaml --device_target Ascend --weight /PATH/TO/WEIGHT.ckpt
Deployment¶
See here.
References¶
[1] Jocher Glenn. YOLOv5 release v6.1. https://github.com/ultralytics/yolov5/releases/tag/v6.1, 2022.